Machine Learning Grants Robots Precise Sense of Touch
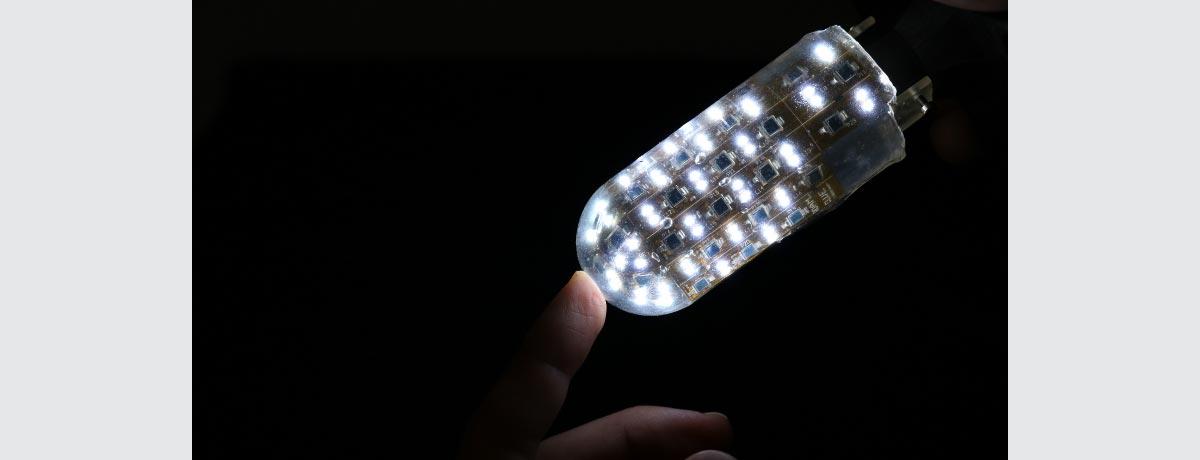
Using both advanced sensor systems and machine learning, Columbia researchers have designed the first robotic finger with a highly accurate sense of touch.
Dexterous robotic manipulation, a robot's ability to handle a variety of objects and actions, relies on gathering and processing a staggering amount of information. Previous methods for building touch robotic fingers have been challenged by the difficulty in covering complex surfaces, and the sheer amount of wires, sensors, and information required.
A team at Columbia Engineering has taken a new approach by mapping signals from light emitters and receivers embedded in a transparent layer that covers the finger. Through the light transported between every emitter and receiver, a rich signal data set emerges that changes in response to touch. Using machine learning techniques, researchers can extract the vital information from all that data to pinpoint where the finger is being touched, what it is touching the finger, how much force is being applied, and more.
The final result is a fully integrated, sensorized robot finger, with a low wire count, built using accessible manufacturing methods and designed for easy integration into dexterous hands. The results may well lead to innovations for manufacturing and logistics, and open up possibilities for robotic assistance in health care and other fields. Learn more.
Make Your Commitment Today